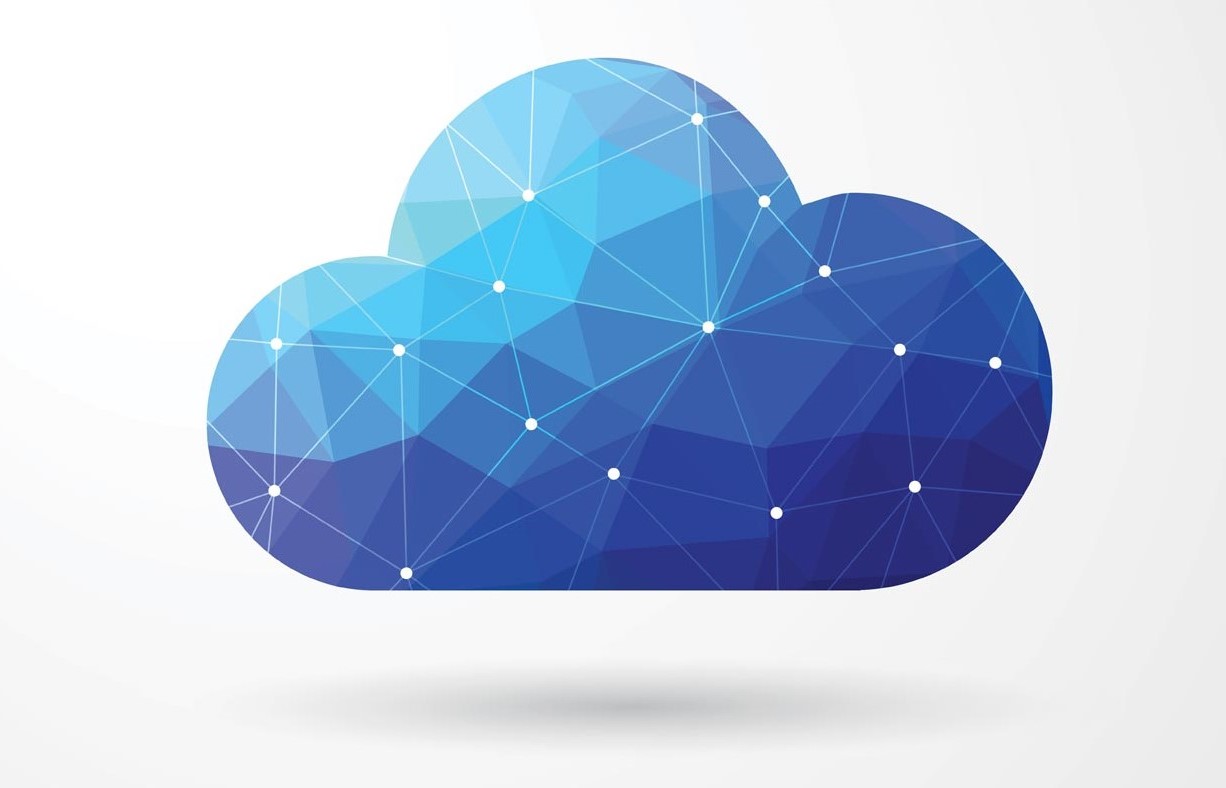
SQL "Google BigQuery" + PYTHON .
Hello Big Data!!! we would like to perform some basic SQL queries using python and bigquery « from google cloud platform.
To get an overview of how to create a google cloud account and use jupyter notebook to run queries there is a detailed documentation here. The free tare grants a massive 1 TB that resets per month, not as massive anyway ,if not careful it takes only a handfull queries to deplete. In this notebook we use a query configuration that if a certain limit is hit ,the query is terminated at that point. some of the datasets that are publicly available on Google BigQuery can be found here.
Data loading.
from google.cloud import bigquery
# Create a "Client" object
client = bigquery.Client()
# Constructing a reference to the "chicago_taxi_trips" dataset
dataset_ref = client.dataset("chicago_taxi_trips", project="bigquery-public-data")
# API request - fetch the dataset
dataset = client.get_dataset(dataset_ref)
# seeing the tables available in the said dataset
tables = list(client.list_tables(dataset))
# looking at the tables in the dataset
for table in tables:
print(table.table_id)
output: taxi_trips
Data overview.
# Constructing a reference to the "full" table
table_ref = dataset_ref.table("taxi_trips")
# API request - fetch the table
table = client.get_table(table_ref)
# looking at the first few elements of the table
client.list_rows(table, max_results = 5).to_dataframe()
id | unique_key | taxi_id | trip_start_timestamp | trip_end_timestamp | c… |
---|---|---|---|---|---|
0 | f7ec1bc4ddd0e39531fd5307fca2a0aa46230451 | 3683df8abf7c59bf0e9d46a0de6f2c4ed65b0971fdaa22… | 2019-05-18 22:30:00+00:00 | 2019-05-18 22:30:00+00:00 | c… |
1 | 96392e56b1f1e528c6538a7f540980ef425757f8 | bc709a696db40a46144faa530198a65442402f42513ee4… | 2013-05-24 14:15:00+00:00 | 2013-05-24 14:15:00+00:00 | c… |
2 | f7e93165a7c38e5f32659b4e29fc9f991201a706 | fd2da3c465274925140779eb37aca0868e129d3d16c76f… | 2019-05-05 22:00:00+00:00 | 2019-05-05 22:15:00+00:00 | c… |
3 | f7e0af3e0673cfd0d8fbe0264d99092ea2d0cfec | 692320c13d5f7819864fb57797a53cd467452176b95411… | 2019-05-09 23:15:00+00:00 | 2019-05-09 23:30:00+00:00 | c… |
4 | f7e9255b6f8b0af6595ed81b0b755f0754d80877 | 88d3be8c1334607f62a8c058f680dd7fbb57826ec7408d… | 2019-05-15 10:45:00+00:00 | 2019-05-15 11:30:00+00:00 | c… |
5 rows × 23 columns
Now that we have seen an overview of the data i.e columns and the way the data is formated. We can investigate the number of trips and get some few insights on the same. The number of trips per year would be a great start.
Some Querying.
rides_per_year_query = """
SELECT EXTRACT(YEAR FROM trip_start_timestamp) AS year, COUNT(1) AS num_trips
FROM `bigquery-public-data.chicago_taxi_trips.taxi_trips`
GROUP BY year
ORDER BY year
"""
# Set up the query (cancel the query if it would use too much of
# your quota i.e 1 gigabyte)
safe_config = bigquery.QueryJobConfig(maximum_bytes_billed=10**10)
rides_per_year_query_job = client.query(rides_per_year_query, job_config = safe_config)
# API request - run the query, and return a pandas DataFrame
rides_per_year_result = rides_per_year_query_job.to_dataframe()
# View results
print(rides_per_year_result)
id | year | num_trips |
---|---|---|
0 | 2013 | 27217716 |
1 | 2014 | 37395436 |
2 | 2015 | 32385875 |
3 | 2016 | 31759339 |
4 | 2017 | 24988003 |
5 | 2018 | 20732088 |
6 | 2019 | 16477365 |
7 | 2020 | 3889032 |
8 | 2021 | 1735699 |
We can try something a bit more interesting. Lets take a look at the number of trips made by the taxis per month in the year 2020.
rides_per_month_query = """
SELECT EXTRACT(MONTH FROM trip_start_timestamp) AS month,
COUNT(1) AS num_trips
FROM `bigquery-public-data.chicago_taxi_trips.taxi_trips`
WHERE EXTRACT(YEAR FROM trip_start_timestamp) = 2020
GROUP BY month
ORDER BY month
"""
# Setting up the query
safe_config = bigquery.QueryJobConfig(maximum_bytes_billed=10**10)
rides_per_month_query_job = client.query(rides_per_month_query, job_config = safe_config)
# API request - run the query, and return a pandas DataFrame
rides_per_month_result = rides_per_month_query_job.to_dataframe()
# Viewing the results
print(rides_per_month_result)
id | month | num_trips |
---|---|---|
0 | 1 | 1073338 |
1 | 2 | 1122124 |
2 | 3 | 557579 |
3 | 4 | 55623 |
4 | 5 | 71660 |
5 | 6 | 102830 |
6 | 7 | 130557 |
7 | 8 | 142879 |
8 | 9 | 162913 |
9 | 10 | 177794 |
10 | 11 | 142678 |
11 | 12 | 149057 |
That is quite interesting, lets spice up things a bit …because why not. Taking hourly number of trips and the average speed in miles per hour in the year 2020 “subset of it”. And to get actual trips that is covered more than ‘0’ miles and more than ‘0’ seconds.
speeds_query = """
WITH RelevantRides AS
(
SELECT EXTRACT(HOUR FROM trip_start_timestamp) AS hour_of_day, trip_miles, trip_seconds
FROM `bigquery-public-data.chicago_taxi_trips.taxi_trips`
WHERE trip_start_timestamp > '2020-01-01' AND
trip_start_timestamp < '2020-07-01' AND
trip_seconds > 0 AND
trip_miles > 0
)
SELECT hour_of_day, COUNT(1) AS num_trips,
3600 * SUM(trip_miles) / SUM(trip_seconds) AS avg_mph
FROM RelevantRides
GROUP BY hour_of_day
ORDER BY hour_of_day
"""
# Set up the query
safe_config = bigquery.QueryJobConfig(maximum_bytes_billed=10**10)
speeds_query_job = client.query(speeds_query, job_config =safe_config)
# API request - run the query, and return a pandas DataFrame
speeds_result = speeds_query_job.to_dataframe()
# View results
print(speeds_result)
id | hour_of_day | num_trips | avg_mph |
---|---|---|---|
0 | 0 | 47224 | 18.973647 |
1 | 1 | 37352 | 17.031103 |
2 | 2 | 28278 | 16.350224 |
3 | 3 | 22949 | 17.524992 |
4 | 4 | 19265 | 22.631116 |
5 | 5 | 20688 | 26.758792 |
6 | 6 | 36174 | 23.027521 |
7 | 7 | 78011 | 17.244791 |
8 | 8 | 123628 | 14.253745 |
9 | 9 | 134458 | 15.204374 |
10 | 10 | 126243 | 17.483727 |
11 | 11 | 137482 | 18.030997 |
12 | 12 | 147606 | 17.720764 |
13 | 13 | 152182 | 17.431356 |
14 | 14 | 152374 | 17.408847 |
15 | 15 | 157111 | 16.296931 |
16 | 16 | 176285 | 14.745277 |
17 | 17 | 192834 | 13.227664 |
18 | 18 | 181006 | 13.409488 |
19 | 19 | 153246 | 16.411717 |
20 | 20 | 124505 | 19.829045 |
21 | 21 | 101503 | 20.169119 |
22 | 22 | 81064 | 19.573663 |
23 | 23 | 61318 | 18.730092 |
We have incooporated common table expression(CTE) in the last part. This makes it a bit more clearer and easier to debug when dealing with a more complex query.